Whether you are from an academic background or not, as an entrepreneur these days you can’t afford to ignore the power of data science. After all, it could be the key to the success of your business, especially if it’s a tech-based one.
Recently we had the honor of hosting Vasilis Vassalos, a professor at the Athens University of Economics and Business (AUEB), as a guest speaker in one of our Friday Q&A sessions. Among other things, Vasilis lifted the lid on all things data science. And told us how he managed to achieve an exit with a startup, almost two decades ago.
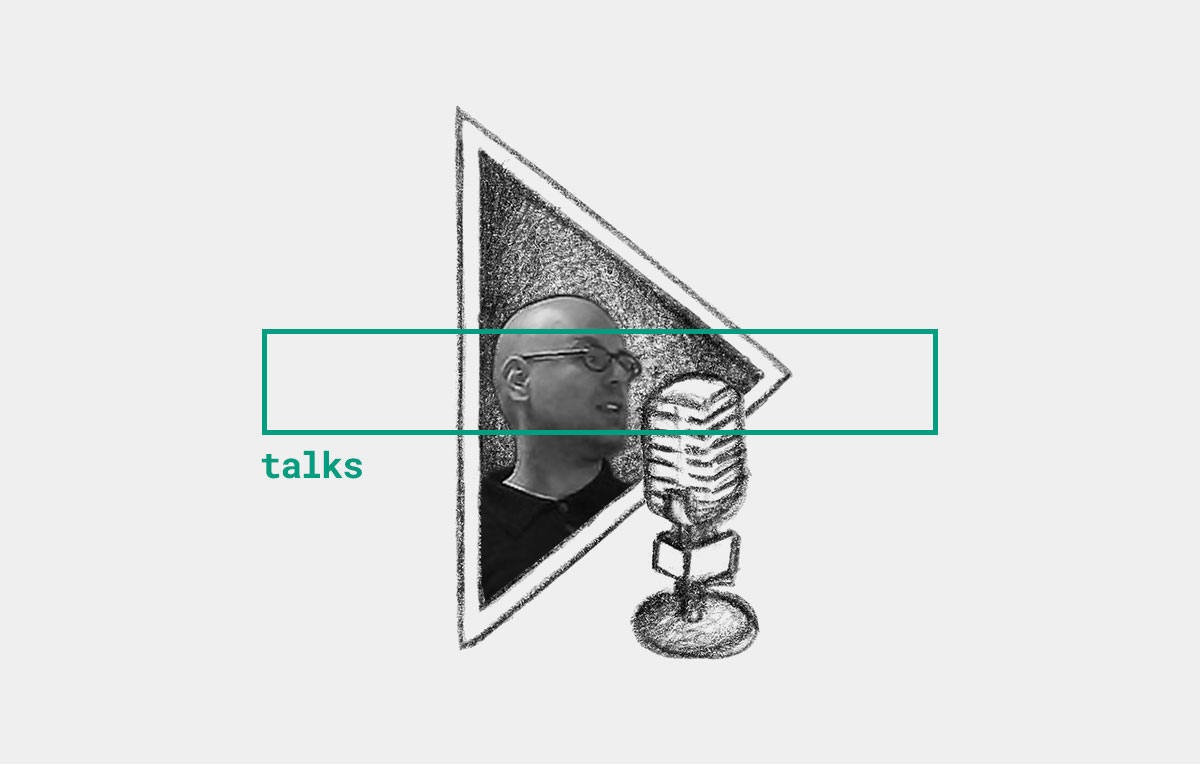
Data science and startups
All of our startups are tech-focused, and almost all of our team members have a background in computing. That’s why we’re more than excited to welcome scientists to our community. This is especially true for guests that bring their in-depth knowledge on specialized fields. They feed our inquiring minds to make our startups better – and help us come up with new business ideas.
Vasilis shared his valuable insights both from the academic and business terrains. His most recent cooperation with Workable as a Vice President of Data Science and his role today as Chief Data Scientist at Velti only add further to his strong scientific background.
A few words about our guest
But Vasilis’ strong academic experience in a such demanding scientific field as Data Science and AI is only one part of his background. He also has a great experience in entrepreneurial environment. This came almost 20 years ago when as a co-founder and CTO of a startup in San Diego, he managed to achieve a great startup success: an exit.
Today, Vasilis leads the Data Science research group in the Information Processing Lab of AUEB. He and his team focus mostly on Data Science life-cycle problems. That’s what most of their projects and publications are all about. A key part is modeling, which is arguably the “sexiest” part of Data Science, as he says. As he explained to us what they do is try to find ways to leverage Data Management; and make, that way, Machine Learning more efficient. Most of the time that is possible with new fundamental, algorithmic ideas.
Obviously, in this post, we don’t have so much time and space to explain Vasilis’ great presentation on Data Science concepts. We can share part of that technical presentation to get a taste.
Scratching the surface of Data Science
When it comes to machine learning problems, such as recommendation problems, it takes more than just adding your data to a recommendation system, in order to get the desired output. As Vasilis explained to us, you need to first adapt your data before you “feed” your system. Or at times, you may even need to go a step further and make a specialized research for that specific problem you wish to solve. And even develop a new method if needed.
More specifically, normalized database tables that represent our conceptual information need to be transformed before proceeding to the next step. In other words, you need to “extract” the appropriate information, in the form of features, to feed a machine learning algorithm. These “feature vectors” contain the transformed input data you’ll need, and are basically a (possibly complex) combination of attributes.
Then, you’ll have to use these vectors as input for your machine learning framework. It could be one of those offered by your ML system or a new one you need to research and develop for this problem. The second case is significantly harder. But it is also, very often, the only way to get an edge in an area with lots of competition.
What is interesting is that, despite their depth and mathematical sophistication, machine learning algorithms and systems mostly deal with files. Input feature vectors are files, and trained models are usually stored as files. The whole information management for Machine Learning is processed using files. While this makes life simpler, it foregoes the many advantages database engines have brought to information management. So, given his background, Vasilis’ research tries to bring the benefits of database processing to ML pipelines. That’s by pushing more of the information processing into database engines.
Now let’s get some details concerning his company’s success back in the early 2000s.
Startup success in the early 00s
As an entrepreneur, Vasilis started in 2000 at the peak of the 1st dot com boom. His company was a pioneer in virtual data integration infrastructure. Their software solution worked as an abstract query layer over different data lakes (this is basically a system or repository of data stored in its natural/raw format, be it text files, spreadsheets, or database tables). The company offered a product that built a unified Database or a “360 view of data assets”, as Vasilis described it, over the data lakes.
The core functionality of their solution gave users the option to integrate multiple channels in marketing, sales, even in accounting and other internal processes of a business. In other words, instead of setting up a data warehouse, moving all these assets into it at great expense and effort, you could alternatively set up a virtual data warehouse very easily. Very cool.
Their enterprise information integrator acted as logic for the data management and data flow. As Vasilis explained to us they targeted mostly customer service applications.
There were other solutions available in this space, but our company was a pioneer in distributed queries over “native” data sources,
he said.
And that was actually what led them to success: his company managed to get an exit at the end of 2003, being sold to a multinational software house (since sold to Oracle). And it was right after this that Vasilis decided to move back to Greece. That’s accepting an offer as a professor at AUEB, also leaving his professor post at the New York University.
As usual, we got the chance to ask Vasilis a few quick-fire questions as we do with all of our guests, and get his words of wisdom as a guide for our future steps.
Q: What made you choose academic environment over an entrepreneurial one?
A: Well, during that period when I was running my startup company, I was also a professor at NYU so I was also part of academia. I would say that although this is not always the case with many startups, it’s really beneficial if one or more of the founders have good experience and track record in both spheres for more technically sophisticated products and services.
Q: What advice would you give to a startup founder?
A: I’ll base my advice on my previous experience. My co-founder and I were both professors during that period, commercializing our cutting-edge research. We both took extended time off our university work to set the company up and get it going. But we both kept our professor positions. And, at times, we tried to juggle both sets of responsibilities. This also meant that we acquiesced to bringing an outsider on board as CEO very early in the company’s development. These weren’t great choices, despite our exit, he admits.
So, first, at least one technical founder needs to be with the company full-time for the duration. And second, a CEO should not be recruited too early from outside the founding team.
Q: When we talk about startup companies, technology and data science, which overlap is the biggest opportunity today?
A: Well, first of all I’d say that you should go for a software product that targets only a few verticals. Your solution should come as a tool that helps customers accomplish things they’ve been craving for in their daily life. One that will help them solve problems in the most efficient way. This means focusing on benefits rather than features. And of course, one that is simple enough to make them adopt it right from the beginning. I believe there’s still a wide margin in the market for ventures like this.
As self-confessed lovers of habit-forming products which are made the lean startup way, we couldn’t agree more, Vasilis.